This is our latest pilot publication, finalized after our Argentina and USA pilots in another domain – offshore. We moved into the Dutch North Sea this time, to test if our methodology can additionally assist in de-risking critical drilling decisions. Biodentify applied and blind tested patented DNA fingerprinting technology and, using advanced machine learning algorithms, accurately predicted hydrocarbon presence in the subsurface using stored cuttings from drilled wells.
The main goal was to check if these cuttings, stored worldwide in core houses and taken at the time the well is drilled, can help train machine learning models and predict new locations. At the new locations seabed samples will be taken prior to drilling, as no cutting material is available with no wells drilled. Since offshore wells are extremely expensive to drill, especially in deep(er) water, it is attractive to use our workflow as a direct hydrocarbon indicator to estimate if prospects are charged or not—thus negating the risk of drilling unproductive wells.
Why the Dutch North Sea
The Dutch North Sea was chosen as an offshore test area because it has seen substantial exploration and production activities over the past 40 years. Additionally, there was already a high-quality online database with well data and a core house filled with stored cuttings from the drilled wells. The data from this project delivered proof of technology, in the form of a highly accurate predictive model of subsurface hydrocarbon productivity, resulting in 82% correct classification of oil/gas vs dry.
Biodentify’s Patented Technology
Biodentify’s patented technology is based on the response elicited in the shallow soil (<30cm) microbial population by hydrocarbons which have migrated from depth to the surface through vertical microseepage. The trace hydrocarbons released are otherwise undetectable, but the bacterial population acts as an extremely sensitive indicator. Samples of 0.15 cm3 are collected from the cuttings and analyzed for microbial DNA content leading to a characteristic fingerprint, from which final predictions can be made. Cutting samples originate from 10-3200 m in depth, in wells drilled between the 1960s-2010s.
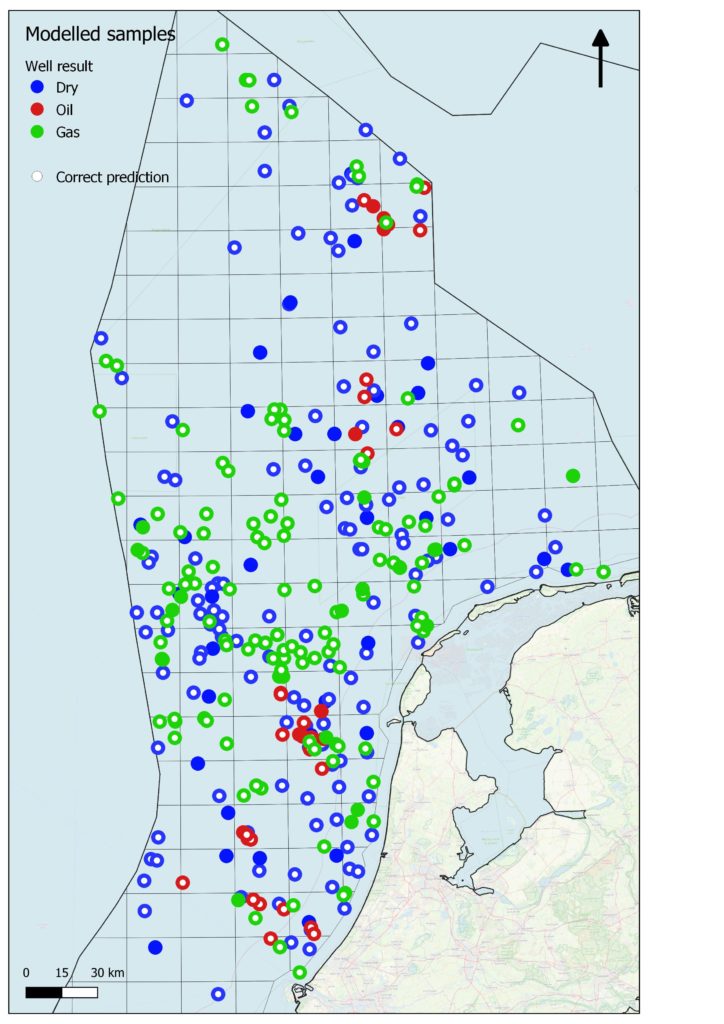
Productivity predicted with cuttings samples using 69 DNA strings as bio-speicies markers. Total accuracy is 82% correct prediction, correct predictions denoted with white dots.
Stored cuttings in core house at Zeist, the Netherlands.
Planning and executing the project with our customers
The project was set up in cooperation with three operators active in the Dutch North Sea. We concentrated on sampling locations with known production statistics, taking a total of 1080 samples. Three different sets of samples were taken: oil producing wells, gas producing wells, and dry wells.
The selected wells were located across the entire Dutch offshore area with a 50/50 distribution of wells that did and did not encounter any oil and or gas. Some wells have samples collected from various depths, which are collected at the Dutch core repository in Zeist. This is managed by TNO/Geological Survey of the Netherlands.
We analyzed the samples for microbes in our lab, separating the microbial DNA from the soil, ending up with a DNA fingerprint for each location. This data is very vast, hundreds of millions of numerical values, too vast for us humans to make sense of it alone. This is where supercomputing power comes in. The use of our unique and constantly updated DNA database, alongside big data algorithms, machine learning and our own metagenomics pipeline, ensure that the model is constantly enhanced and improved over time to enable us to accurately predict subsurface hydrocarbon presence.
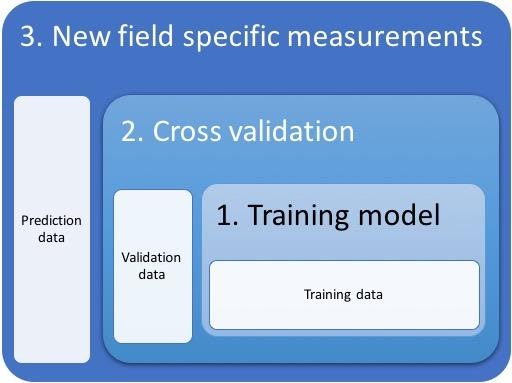
Three categories of DNA/productivity data from the cutting samples: 1) training data used to build a model, 2) validation data to test if the model is accurate enough and 3) prediction data to test the prediction of 100 cuttings totally left out when model building.
Firstly 100 samples were set aside to use in prediction after training the model; the remaining samples were used to perform the training. For building a maximally predictive model, we use three loops, all making use of different data areas.
- Inner loop/training: 70% of the available training samples are used to find a correlation which is shown in the innermost loop of the above figure.
- Second loop/cross validation: After constructing a predictive model, it is tested by cross-validation on the 30% left out of the training samples, shown in the second loop of the above figure. This is randomly repeated 100 times. Therefore, cross validation of the 100 predictive models can also be made 100 times. This leads to a total of 10,500 predictions from our samples, which means every sample will be left out on average 30 times. Statistics of these cross-validations are then made via scoring the number of times that a prediction is right vs wrong.
- Third loop/test: Within these 100 models, predictions can be made on the 100 test samples that were set aside, shown in the outer loop of the above figure. This is done 10 times with different random chosen test sets. Results of these predictions have been shown to be 82% accurate, on average, for the 10 test sets (see first figure above). In the case of an over fitted model, we would see good calibration but bad predictions.
Testing causality of the models
The high accuracy obtained means that there is a significant difference between the DNA fingerprints of oil and gas wells versus those of dry wells. This can be seen as statistical proof for microseepage. To link the model to microseepage by causality, we investigated what is known about the microbes selected by the machine learning model. These selected microbes have been well investigated in scientific literature.
Scientific research performed worldwide sees relationships between the degradation of oil and gas and abundances of certain microbes. We can clearly see that the microbes found by the machine learning model which are found above oil and gas wells are described in literature as dominantly degrading hydrocarbons for their metabolism (hydrocarbon lovers). Vice versa that microbes which are more abundant above dry wells by the machine learning model find hydrocarbons toxic (hydrocarbon haters) concluded from independent scientific research.
Selected microbes in the model above oil and gas wells (left figure) vs selected microbes above dry wells (right figure) and their link to literature. The size of the contribution to the pie chart microbe indicates the weight the microbe gets in the model (measured for bio-species marker importance).
Conclusions and future work
The high accuracy of differences in DNA fingerprints from cuttings above oil and gas wells versus dry wells gives us great confidence that we can use these samples, stored in national or operator run core houses, to de-risk new well locations above prospects. Although this is an exciting and cost effective way of prediction, new areas do not have cuttings available. Because of this we need to be able to predict hydrocarbon presence based on seabed samples.
Next step is to link the results from cuttings samples to seabed samples. This has to be systematically tested with a large seabed sample set. Currently this exercise is being discussed for the Dutch North Sea. Additionally, we are in proposal discussions on an extended seabed survey with a number of operators in the Norwegian Continental Shelf. This small consortium is still open for other operators to join – more on this will come out shortly but please reach out to us if you are keen to hear more on this exciting opportunity.
About Biodentify
Biodentify was founded as a spin-out from Dutch R&D group TNO in December 2014. TNO is a 3000+ R&D organization and the largest microbiological research group in the Netherlands. Biodentify is owned and managed by three partners with extensive entrepreneurial experience bringing innovative new technologies to market in the Oil & Gas industry. The company has developed a novel and patented technology, predicting prospectivity before drilling, with > 70% accuracy, based on microbial DNA analysis of shallow soil or seabed samples through unique machine learning models. Biodentify was named the #58 most innovative company in 2019 by the KvK (Chamber of Commerce).